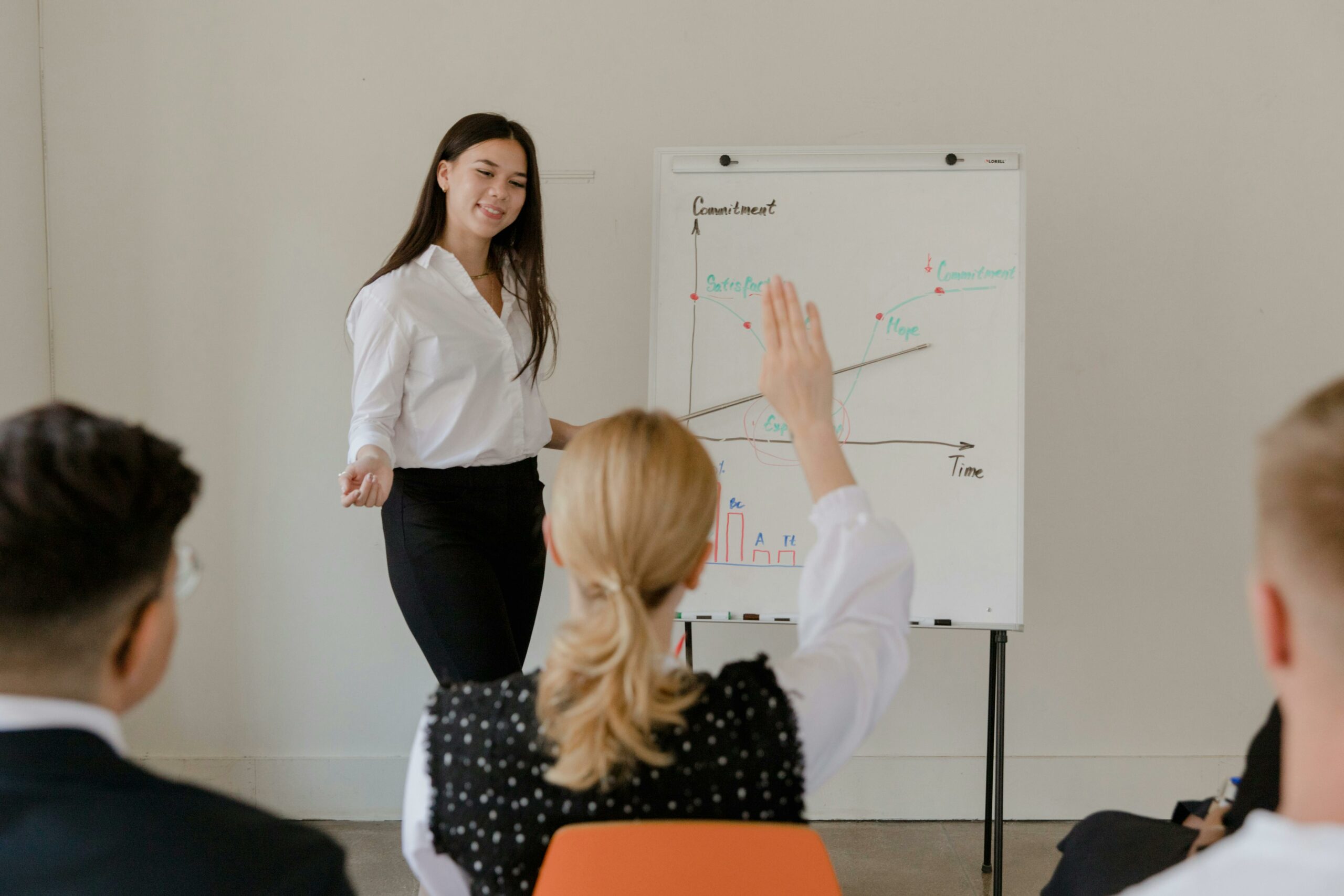
Getting your Trinity Audio player ready...
|
Researchers often have to draw statistical conclusions about a population based on a smaller sample of data. For this kind of statistical inference, they use probability distributions. These are of various types: binomial distribution, cumulative distribution, etc. This article will dive into the Poisson distribution, and its uses in research.
See also: 6 Top questions from Editage’s recent webinar “Unpacking Data Distributions”
What is a Poisson distribution?
A Poisson distribution is a probability distribution used for discrete (= countable) data. A Poisson distribution tells you the probability of a countable outcome, like how many deaths will occur in a group of elderly nursing home residents in a year.
Key assumptions
A Poisson distribution isn’t always applicable for your data or study. You need to fulfill the following conditions:
- Events happen at random: There is no pattern or predictability in the number of events. So, if you’re measuring the number of hypothermia cases in a year, you can’t use the Poisson distribution because the number of cases obviously clusters in and peaks in winter.
- Individual events are independent of each other. This condition means that the probability of one event doesn’t affect the probability of the other. For example, if you’re measuring the number of stroke episodes in a group of people, a Poisson distribution might not be applicable because having a stroke increases your chances of having another stroke episode.
- You know the mean number of events occurring within the given time period. This number is called λ (Greek letter lambda). In a Poisson distribution, λ is assumed to be constant.
- Events are rare: The Poisson distribution assumes that the frequency of events is small and that events are spread out, i.e., not clustered together. Also, if the events happened frequently, it’s likely that they aren’t actually independent of each other (see point 1).
Mean, variance, and lambda
Usually, any probability distribution has a mean and a variance. However, for a Poisson distribution, the mean and the variance are the same. They’re denoted by λ.
What is the difference between normal distribution and Poisson distribution?
The most commonly used type of probability distribution is the normal distribution (the Gaussian bell curve). It’s used for continuous data (data can take on an infinite number of values) like height, weight, etc. In contrast, the Poisson distribution works only for count data, where the data can take the values of non-negative integers only: gravida, number of falls, number of ischemic strokes, etc. These can’t be fractions or negative numbers.
Advantages and uses in research
- Simplicity: The Poisson distribution is mathematically simple and easy to apply
- Flexibility: It can be used in a variety of scenarios and research fields.
If you want further examples of how the Poisson distribution can be used, see how Yu et al. (2023) used Poisson distributions to model the lifetime incidence of cancer in people. Mubarik et al. (2023) similarly used a Poisson distribution to model breast cancer outcomes. Acuna-Hidalgo et al. (2017) also used a Poisson distribution in their study on the prevalence of clonal hematopoiesis-associated mutations.
Leave a Reply